Can AI be Meaningfully Regulated, or is Regulation a Deceitful Fudge?
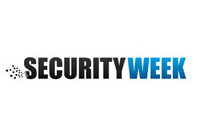
Wednesday, July 10, 2024
Both models have strengths and weaknesses, and both models have their share of failures. GDPR, for example, is often thought to have failed in its primary purpose of protecting personal privacy from abuse by Big Tech.
Ilia Kolochenko, chief architect and CEO at ImmuniWeb, attorney-at-law with Platt Law LLP, and adjunct professor of cybersecurity & cyber law at Capitol Technology University, goes further. “I’d be even more categorical,” he says. “GDPR is foundationally broken. It was created with good and laudable intent, but it has failed in its purpose. Small and medium businesses are wasting their time and resources on pseudo-compliance; it is misused as a method of silencing critics; and large companies and Big Tech are not conforming to it.”
The basic problem for GDPR is that individual users have ineffective recourse to redress – Big Tech can simply throw resources (money and lawyers) at the problem. “They hire the best lawyers to intimidate both the plaintiffs and the authorities, and they send so many documents the plaintiff abandons the complaint or settles for a very modest amount,” continued Kolochenko.
The danger is that the EU’s recent monolithic AI Act will go the same way as GDPR. Kolochenko prefers the US model. He believes the smaller, more agile method of targeted regulations used by US federal agencies can provide better outcomes than the unwieldy and largely static monolithic approach adopted by the EU.
He is not alone in believing that sector-specific regulation would be better than a ‘one-size fits all approach’. In Truly Risk-Based Regulation of Artificial Intelligence published on June 16, 2024, Martin Ebers (Professor of IT Law at the University of Tartu, Estonia) writes: “Regulators should tailor regulations based on the specific risks associated with different AI applications in various sectors.”
Kolochenko believes the US model provides both risk-based regulation and better support for end users.
Of course, agency-based regulations are not perfect – consider the current concerns over the SEC disclosure rules – but he believes they can and do rapidly improve. He points to the effect of the LabMD case against the FTC. The FTC sought to require a complete overhaul of LabMD’s data security following breaches in 2005 and 2012. LabMD appealed; and the court ruled that the FTC couldn’t require a complete security overhaul without specifying the exact inadequacies of LabMD’s practices.
“Since then, the FTC has effectively increased its technical and legal teams” continues Kolochenko. “Now, if you read their settlements, you see training, data minimization, penetration testing, vulnerability scanning, backups, resilience – all kinds of details.” Agency rules are inherently easier to adapt to evolving circumstances than monolithic laws.
“I guess with the SEC we’ll have a similar source of knowledge soon. Honestly, I don’t think it will be a big challenge to define ‘a material cybersecurity incident’. It will be doable. I suspect that defining the requirements for AI rules will be equally doable.” And equally adaptable going forward.
The big difference for Kolochenko is that with the AI Act (and GDPR), the wronged must go to court and prove their case against mega-rich companies; while the US model requires each individual company, large or small, to state effectively under oath and subject to personal legal repercussions: “We have done no wrong.” It’s a question of reversing the onus. Lying to the agency could lead to criminal liability for wire fraud.
Is it already too late for effective regulation?
AI is already here, and it is moving faster than legislators can legislate. Since retrospective (or retroactive) legislation is disfavored if not disallowed, new regulation is based on the regulators’ assumption of the future developments and use of AI. This explains why the AI Act concentrates on the inference (or use) rather than the creation of gen-AI models – the models already exist, the data used to train them has already been ‘stolen’.
“I think this is the biggest robbery in the history of humanity,” comments Kolochenko. “What the big gen-AI vendors did was simply scrape everything they could from the internet, without paying anyone anything and without even giving credit.” Arguably, this should have been prevented by the ‘consent’ elements of existing privacy regulation – but it wasn’t.
Once scraped it is converted into tokens and becomes the ‘intelligence’ of the model (the weights, just billions or trillions of numbers). It is effectively impossible to determine who said what, but what was said is jumbled up, mixed and matched, and returned as ‘answers’ to ‘queries’. The AI companies describe this response as ‘original content’. Noam Chomsky describes it as ‘plagiarism’, perhaps on an industrialized scale. Either way, its accuracy is dependent upon the accuracy of existing internet content – which is frequently questionable. Read Full Article
IT PRO: Microsoft has told workers in China to ditch Android and use iPhones – here’s why
SecurityWeek: Supreme Court Ruling Threatens the Framework of Cybersecurity Regulation